Type I Error vs. Type II Error: What's the Difference?

Edited by Aimie Carlson || By Janet White || Published on February 2, 2024
A Type I error occurs when a true null hypothesis is incorrectly rejected, while a Type II error happens when a false null hypothesis is incorrectly accepted.

Key Differences
A Type I error, also known as a false positive, involves rejecting the null hypothesis when it is actually true. This error leads to the assumption that there is an effect or difference when there isn't one. In contrast, a Type II error, or false negative, occurs when the null hypothesis is not rejected despite being false, leading to a missed detection of an actual effect.
The probability of making a Type I error is denoted by alpha (α), which is typically set at 0.05 in many studies. This means there's a 5% chance of rejecting the null hypothesis when it is true. On the other hand, the probability of making a Type II error is denoted by beta (β), and the power of the test (1 - β) is the probability of correctly rejecting a false null hypothesis.
In the context of a medical trial, a Type I error might involve concluding that a new drug is effective when it’s not, potentially leading to unnecessary treatments. A Type II error, however, would occur if the trial concludes that the drug is not effective when it actually is, missing out on potential benefits.
The consequences of Type I and Type II errors can be vastly different. A Type I error might lead to unwarranted actions or changes in belief, whereas a Type II error represents a missed opportunity or lack of action when one is needed. The seriousness of either error depends on the specific context and the potential impact of incorrect conclusions.
Balancing these errors is crucial in hypothesis testing. Minimizing a Type I error might increase the risk of a Type II error and vice versa. Researchers must decide the acceptable levels of these errors based on the relative importance of false positives and false negatives in their specific context.
ADVERTISEMENT
Comparison Chart
Definition
False positive; rejecting a true null
False negative; accepting a false null
Symbol
Alpha (α)
Beta (β)
Example Context
Declaring a non-effective drug as effective
Failing to recognize an effective drug
Consequence
Unwarranted action/change in belief
Missed detection/opportunity
Relation to Hypothesis Testing
Probability of incorrectly rejecting null
Probability of incorrectly accepting null
ADVERTISEMENT
Type I Error and Type II Error Definitions
Type I Error
Leads to unnecessary actions based on incorrect conclusions.
A company recalling a safe product due to a Type I error leads to unnecessary costs.
Type II Error
Failing to reject a false null hypothesis.
A Type II error occurs in a study that fails to detect the benefits of a new medicine.
Type I Error
Occurs when an effect is assumed where none exists.
A Type I error happened when a study incorrectly showed a diet causing weight loss.
Type II Error
Results in missed opportunities or lack of necessary action.
Not investing in a profitable venture due to a Type II error means missing out on gains.
Type I Error
Rejecting a true null hypothesis.
Declaring a new treatment effective when it's not is a Type I error.
Type II Error
Occurs when a real effect is overlooked.
A Type II error was made when a test failed to show the impact of pollution on health.
Type I Error
Known as a false positive error.
Finding an innocent person guilty is a classic Type I error in legal trials.
Type II Error
Beta (β) represents the probability of this error.
A β of 0.20 in a study indicates a 20% chance of making a Type II error.
Type I Error
Alpha (α) represents the probability of this error.
Setting α at 0.05 means a 5% risk of making a Type I error.
Type II Error
Known as a false negative error.
Missing a diagnosis in a patient who is actually sick is a Type II error.
FAQs
Can both Type I and Type II errors occur in the same test?
No, they are mutually exclusive in a single hypothesis test.
How is a Type II error different from a Type I error?
A Type II error is incorrectly accepting a false null hypothesis, a false negative.
What symbol represents the probability of a Type I error?
Alpha (α).
What is a Type I error?
Incorrectly rejecting a true null hypothesis, a false positive.
How can the risk of a Type I error be reduced?
By setting a lower alpha level, though this may increase the risk of a Type II error.
How does sample size affect Type II errors?
Increasing the sample size can reduce the risk of Type II errors.
Does a high alpha (α) increase the risk of Type I errors?
Yes, a higher α means a greater chance of rejecting a true null hypothesis.
What is the impact of a Type II error in clinical trials?
It could mean failing to recognize the effectiveness of a beneficial treatment.
How is the alpha level chosen in hypothesis testing?
It's chosen based on the acceptable risk level for a Type I error, often 0.05.
Is a Type II error also known as a false negative?
Yes, it's when a false null hypothesis is not rejected.
What does beta (β) represent in the context of Type II errors?
The probability of making a Type II error.
What are the consequences of a Type I error?
Unnecessary actions or changes in belief based on incorrect assumptions.
Are Type II errors more common in studies with small sample sizes?
Yes, small sample sizes often lead to a higher risk of Type II errors.
Is a Type I error always a bad outcome?
It's undesirable, but the severity depends on the context and potential consequences.
What is the significance level in the context of Type I error?
It's the alpha level, indicating the threshold for rejecting the null hypothesis.
Can a Type II error be decreased without affecting Type I error?
Yes, by increasing the sample size or test power, without changing α.
Can improving test sensitivity reduce Type II errors?
Yes, higher sensitivity can help in correctly identifying true effects.
Do Type I errors imply research misconduct?
Not necessarily; they can occur even with proper research conduct.
Why is balancing Type I and II errors important?
To ensure a fair trade-off between the risks of incorrect conclusions.
Why is it impossible to eliminate both error types simultaneously?
Decreasing one usually increases the other; they are inversely related.
About Author
Written by
Janet WhiteJanet White has been an esteemed writer and blogger for Difference Wiki. Holding a Master's degree in Science and Medical Journalism from the prestigious Boston University, she has consistently demonstrated her expertise and passion for her field. When she's not immersed in her work, Janet relishes her time exercising, delving into a good book, and cherishing moments with friends and family.
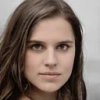
Edited by
Aimie CarlsonAimie Carlson, holding a master's degree in English literature, is a fervent English language enthusiast. She lends her writing talents to Difference Wiki, a prominent website that specializes in comparisons, offering readers insightful analyses that both captivate and inform.
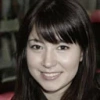