Multicollinearity vs. Autocorrelation: What's the Difference?

By Harlon Moss || Updated on May 20, 2024
Multicollinearity occurs when predictor variables in a regression model are highly correlated, while autocorrelation occurs when residuals in a time series model are correlated over time.
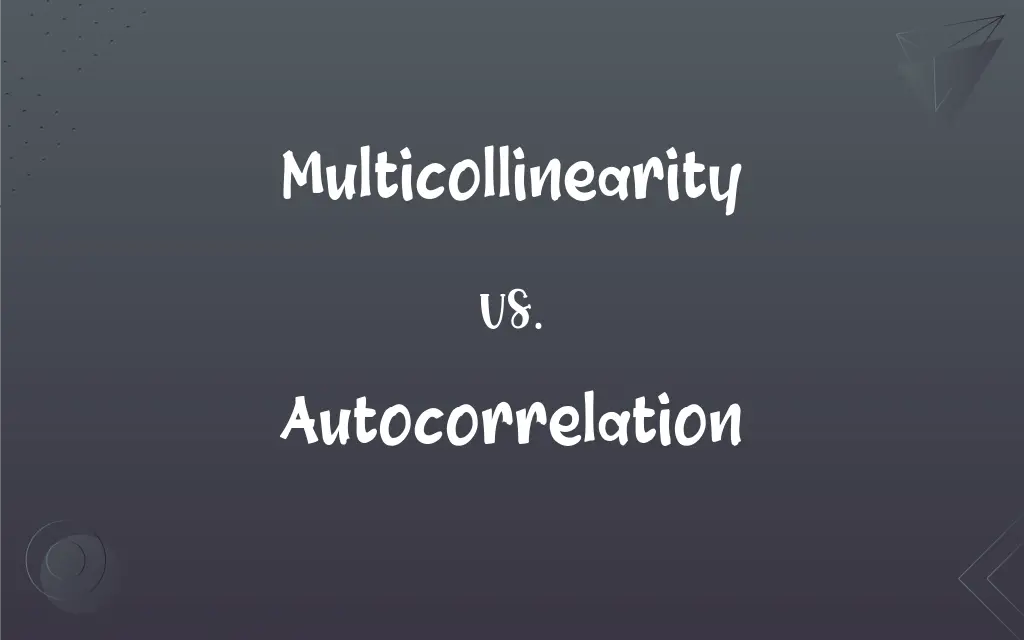
Key Differences
Multicollinearity refers to a situation in regression analysis where independent variables are highly correlated with each other. Autocorrelation, on the other hand, is the correlation of residuals in a time series model across different time periods.
In the context of regression analysis, multicollinearity can be identified through variance inflation factors (VIFs) or correlation matrices, while autocorrelation can be detected using the Durbin-Watson test or autocorrelation function (ACF) plots.
Both multicollinearity and autocorrelation can lead to misleading statistical inferences, but they affect different aspects of the regression model. Multicollinearity impacts the stability and interpretation of coefficients, whereas autocorrelation affects the efficiency and validity of the model's predictions.
Addressing multicollinearity often involves dropping or combining correlated variables, whereas addressing autocorrelation may require adding lagged variables or using more sophisticated time series models.
Comparison Chart
Definition
High correlation among independent variables
Correlation of residuals over time
ADVERTISEMENT
Context
Regression analysis
Time series analysis
Detection
Variance Inflation Factor (VIF), correlation matrix
Durbin-Watson test, autocorrelation function (ACF)
Impact
Inflates standard errors, unreliable coefficients
Inefficiency, invalid predictions
Solutions
Drop/combine variables, ridge regression
Add lagged variables, use ARIMA models
Multicollinearity and Autocorrelation Definitions
Multicollinearity
High correlation among predictors in a regression model.
Multicollinearity makes it difficult to determine the impact of each variable.
ADVERTISEMENT
Autocorrelation
Can lead to invalid statistical inferences.
Autocorrelation invalidated the standard error estimates.
Multicollinearity
Detected using VIF.
The VIF indicated significant multicollinearity between the predictors.
Autocorrelation
Detected using the Durbin-Watson test.
A Durbin-Watson test revealed significant autocorrelation.
Multicollinearity
Can cause unreliable coefficient estimates.
The coefficients were unstable due to multicollinearity.
Autocorrelation
Correlation of residuals over time in a time series model.
Autocorrelation suggests that the residuals are not independent.
Multicollinearity
Leads to inflated standard errors.
The presence of multicollinearity increased the standard errors of the estimates.
Autocorrelation
Indicates model inefficiency.
The model's inefficiency was due to autocorrelation.
Multicollinearity
May require variable elimination or transformation.
To address multicollinearity, we removed the highly correlated predictors.
Autocorrelation
May require adding lagged variables.
We added lagged variables to address the autocorrelation in the model.
Multicollinearity
(statistics) A phenomenon in which two or more predictor variables in a multiple regression model are highly correlated, so that the coefficient estimates may change erratically in response to small changes in the model or data.
Autocorrelation
The cross-correlation of a signal with itself: the correlation between values of a signal in successive time periods.
Multicollinearity
A case of multiple regression in which the predictor variables are themselves highly correlated
FAQs
How does multicollinearity affect regression analysis?
Multicollinearity inflates standard errors and makes coefficient estimates unreliable.
What is multicollinearity?
Multicollinearity is when predictor variables in a regression model are highly correlated.
How can you detect multicollinearity?
Multicollinearity can be detected using the Variance Inflation Factor (VIF) or a correlation matrix.
What is a common solution for autocorrelation?
Adding lagged variables or using ARIMA models are common solutions for autocorrelation.
What does a low Durbin-Watson statistic indicate?
A low Durbin-Watson statistic indicates positive autocorrelation in the residuals.
What is autocorrelation?
Autocorrelation is the correlation of residuals in a time series model across different time periods.
What is a common solution for multicollinearity?
Common solutions include dropping or combining highly correlated variables or using ridge regression.
Why is multicollinearity problematic?
It causes instability in coefficient estimates, making it hard to interpret the effects of predictors.
How does autocorrelation affect time series analysis?
Autocorrelation leads to inefficiency and invalid statistical inferences in the model.
How can you detect autocorrelation?
Autocorrelation can be detected using the Durbin-Watson test or an autocorrelation function (ACF) plot.
Can multicollinearity be present in time series data?
Yes, multicollinearity can occur in time series data if predictor variables are highly correlated.
Can autocorrelation be resolved by differencing the data?
Yes, differencing the data can help resolve autocorrelation in time series analysis.
Does autocorrelation imply causation?
No, autocorrelation does not imply causation; it merely indicates a pattern over time.
Why is autocorrelation problematic?
It indicates that residuals are not independent, leading to inefficient and potentially invalid predictions.
Is multicollinearity more common in small or large datasets?
Multicollinearity can occur in both small and large datasets, but it's more problematic in small datasets due to limited variance.
Is multicollinearity related to overfitting?
Multicollinearity can contribute to overfitting by inflating the variance of coefficient estimates.
Is autocorrelation related to seasonality?
Yes, autocorrelation can be related to seasonality if residuals are correlated at regular intervals.
Can autocorrelation affect non-time series data?
Autocorrelation specifically affects time series data where residuals are correlated over time.
Does multicollinearity affect all regression models?
Multicollinearity affects linear regression models but can also impact other types of regression models.
What does a high VIF indicate?
A high VIF indicates a high level of multicollinearity among predictors.
About Author
Written by
Harlon MossHarlon is a seasoned quality moderator and accomplished content writer for Difference Wiki. An alumnus of the prestigious University of California, he earned his degree in Computer Science. Leveraging his academic background, Harlon brings a meticulous and informed perspective to his work, ensuring content accuracy and excellence.
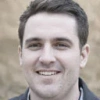